
Our Investment in Datology
Over the past decade, we’ve witnessed several AI booms, driven by breakthroughs in hardware and/or algorithms. While the catalyst for these booms can be unpredictable, the arc of these booms is easier to anticipate: companies realize that they must apply AI to their products to stay competitive and race to release AI apps. When most AI apps fail to meet expectations, it becomes evident that these teams must make more significant investments in data and data management. While many teams shift focus to data quality, they soon realize that building the best datasets is really, really hard.
It’s only been 14 months since chatGPT was released, but we’ve already seen this story play out. Following the successful launch of chatGPT, companies rushed to develop LLM-driven features using commercial LLM APIs and clever prompting strategies. However, within months, many discovered that the only way to achieve their product goals was to pre-train or fine-tune models on their own datasets. And then they got stuck.
In the past decade, many new data tools and platforms have helped practitioners collect, store, organize, and monitor their datasets. These tools have empowered developers to build amazing data and AI-powered applications using smaller, structured datasets. However, none of these tools address the unique needs of generative AI (genAI) developers today. Specifically, genAI developers, most of whom are working with large, unstructured, and unlabeled datasets; are confronted with questions like: How do we remove duplicate or redundant data? What data points are difficult or even harmful? How can we construct and sequence batches?
Datology is the tool that all AI teams need to build products that delight users, minimize costs, and trump the competition. It’s the only available tool that makes data curation easy for genAI developers. Specifically, Datology is a fully automated data curation platform that enables users to train better models for lower cost.
Given the urgent need for tools to curate foundation model pretraining and/or fine-tuning datasets, you might wonder why Datology is the only automated, scalable platform on the market. Well, building a system like Datology is excruciatingly challenging - but the Datology team is exceptional. Not only do they have exquisite taste in investors, but they’re also among the only researchers in the world who know how to solve the technical challenges associated with fully automated data curation, sequencing, and batch construction. In 2022, Datology CEO Ari Morcos published a seminal paper wherein he demonstrated that neural networks can achieve better performance without the need for excessive data or computational resources through careful data pruning. Since then, Datology team members have published research on several other techniques that enable genAI developers to exceed power-law scaling and have designed a platform that is easy to deploy, secure by design, and massively scalable.
We invested in Datology because they have the right team to build a platform that could unlock so many breakthroughs in genAI-driven product development. However, our journey to investing started long ago.
Last year, we had the same conversation with numerous LLM teams. While most used LLM APIs for rapid prototyping, nearly all engineering and ML leaders with whom we spoke planned to adopt self-hosted models to control their product roadmap and leverage proprietary, internal data. Throughout 2023, many organizations released OSS models that matched the performance of LLMs from vendors like OpenAI and Anthropic. However, the biggest obstacle to transitioning to such models was finding the right dataset upon which to fine-tune or pretrain. Based on these conversations, we started meeting researchers who focused on data curation for LLMs. Although this pain point was SO clear, very few researchers wanted to spend hours looking through the Pile to come up with better ideas for data filtering and selection.
In April, Jonathan Frankle, the Chief Scientist of Mosaic, sent us a well-timed message. He offered to connect us with a leading empirical researcher focused on assessing and improving data quality for deep learning. We immediately met with Ari Morcos to discuss the possibility of building “Mosaic for data.” In the following weeks, we introduced Ari to several practitioners who confirmed that this platform would be a “no-brainer” to buy. Many expressed excitement that Datology could help them transition off LLM APIs and dramatically reduce their training costs while improving model performance.
During this time, Ari recruited an incredible co-founder - Matthew Leavitt, with whom he had previously worked at FAIR. Matthew wowed us with his Seinfeld references but also impressed us with his experience intervening on training data from FAIR and Mosaic (where he was previously Head of Data Research) and his commitment to rigorous research and product development. It was clear that Matthew was the right co-founder to challenge Ari to think, grow, and take action.
In late June, we reconnected with Bogdan Gaza, the former founder of Moonsense, who was beginning to search for his next role. Bogdan told us that he was not interested in joining a startup as a co-founder again so soon, but offered to chat with Ari about his experiences building natural language and search infrastructure at Twitter. After just a few meetings, Bogdan knew he had to join. It was evident that Ari and Matthew’s plans to accelerate generative AI-driven product development through data curation were solid - but also that they needed someone with his unique skillset in data and backend infrastructure to execute this vision.
Since we invested, the Datology team has already recruited exceptional researchers and engineers; implemented and validated key algorithms to eliminate duplicate and redundant data; and engaged their first design partners. But what impresses us most is the seamless teamwork among Ari, Matthew, and Bogdan. Their complimentary skills and backgrounds position them perfectly to tackle challenging technical problems and realize their ambitious vision.
We believe that this AI boom is more than hype. We think current and future AI products will radically change how we live and work. However, AI app developers will need better tools to unlock these breakthroughs. The Datology team, led by a stellar group of thinkers and doers, not only addresses the pressing need for scalable data curation and management platforms, but also exemplifies how researchers and engineers can collaborate to unlock AI innovation. We’re thrilled to be their first investor and to support them as they help unleash the full potential of AI.
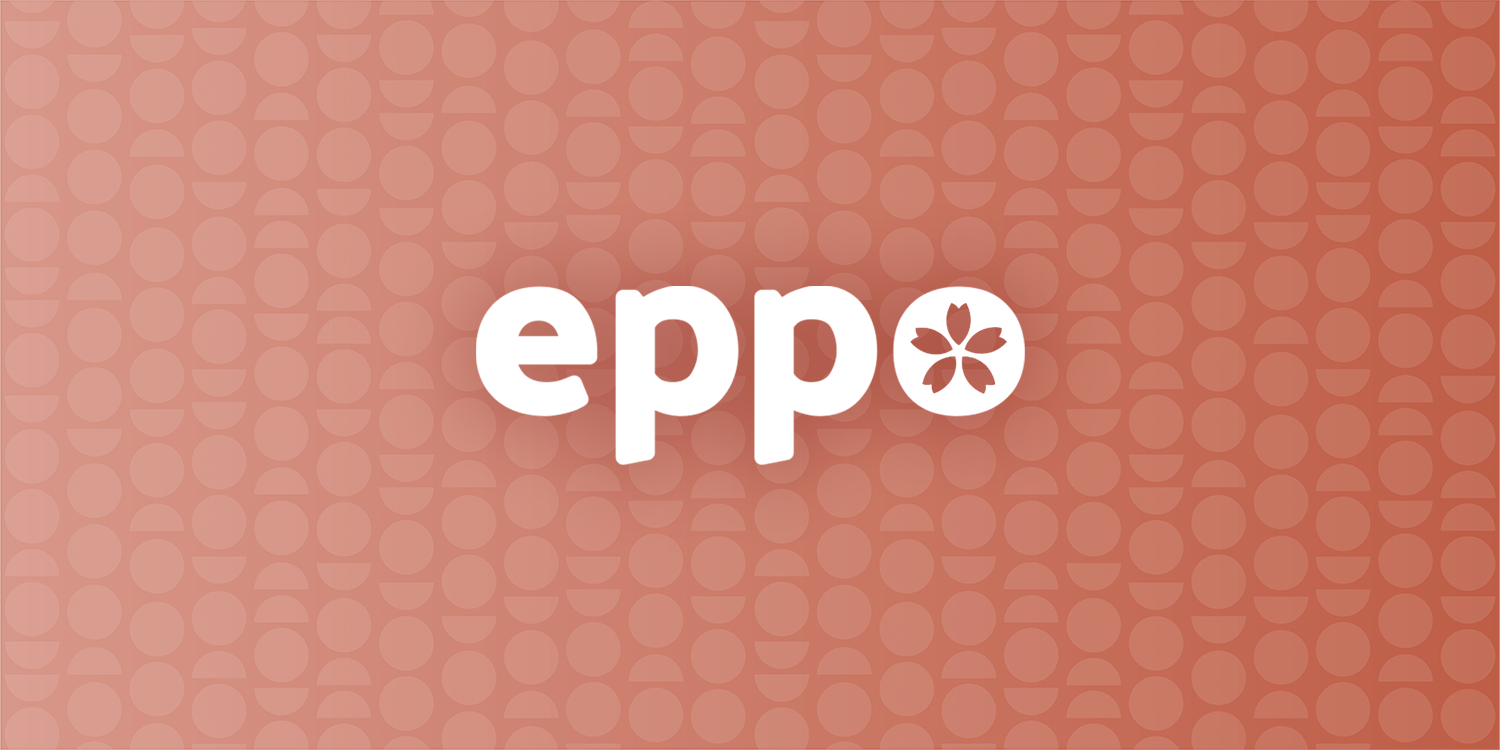
Our Investment in Eppo
Eppo is the tool that every company should use in their experimentation stack – which is why we’re thrilled to announce our seed and Series A investment in the company.
By Natalie Vais
June 22, 2022

Our Investment in Gantry
With a platform like Gantry, ML teams can finally build great ML products quickly and reliably. They can ship ML-driven applications sooner – knowing that they can rapidly integrate user and other feedback that will drive performance gains.
By Sarah Catanzaro
June 7, 2022